Table of Contents
- 1 Impact on society and fake detection technology
- 1.1 Incidents involving deepfakes
- 1.2 Chief Cabinet Secretary fake image spread incident
- 1.3 Papers on breaking eKYC
- 1.4 Fake video detection technology
- 2 Social implementation of generative deep learning
- 2.1 NVIDIA – Maxine
- 2.2 Descript – Overdub
- 2.3 Generated Media – Generated Photos
- 2.4 Artificial tooth design by GAN
- 2.5 Application to design and art
- 2.6 Application to industrial product inspection
- Summary
1 Impact on society and fake detection technology
1.1 Incidents involving deepfakes
The evolution of Deep Learning technology has made it possible for people without advanced technology to create fictitious videos and images as long as they can program and operate a PC to a certain level.
While the democratization of technology has made it possible for individuals to engage in creative activities that were previously difficult to do, it is also possible to engage in activities that demean some people, such as making unauthorized pornographic videos of celebrities. Vicious incidents and crimes have also occurred. Here, we introduce some of them.
1.1.1 Pornographic video incident on Reddit
In December 2017, a Reddit user with the handle “Deepfakes” posted several pornographic videos on the Internet [1]. It was based on actresses and artists, and had a great impact on society, but it soon turned out to be a fake. The incident also sparked the spread of the term “deepfake”.
1.1.2 Energy company CEO impersonation incident
In March 2019, a UK energy company was defrauded millions of dollars by voice deepfake technology. The CEO of this energy company was requested by the CEO of the parent company in Germany to transfer 220,000 euros (approximately 26 million yen) over the phone as soon as possible.
It turned out that it was an instruction from the fraudster who used it. According to the insurance company that investigated this incident, it was reported that the accent and pronunciation of German speakers were reproduced, and they did not realize that it was a synthesized voice[2].
1.1.3 Japanese fake porn video incident
In October 2020, a university student in Kumamoto City and a system engineer in Hyogo Prefecture were arrested on suspicion of posting a pornographic video that synthesized the faces of celebrities on the Internet using deepfake technology [3].
It is said that 30,000 images per video were used for training, and the model was trained for about a week. The case is believed to be the first criminal case in which deepfake technology has been exploited in Japan.
1.1.4 Cheerleader fake video incident in Pennsylvania
On March 10, 2021, in Pennsylvania, the team used deepfake technology to fabricate photos and videos of the daughter of a high school student working as a cheerleader, drinking and smoking naked in order to trick her teammate. The mother who sent it to Mate and Coach was arrested [4].
Additionally, the district attorney charged that the mother sent anonymous messages to her victim, her daughter’s teammate, encouraging her to commit suicide. Her daughter had no knowledge that her mother had committed such a crime, according to reports citing her sentence.
1.2 Chief Cabinet Secretary fake image spread incident
When an earthquake with a seismic intensity of upper 6 occurred in Fukushima and Miyagi prefectures in February 2021, then Chief Cabinet Secretary Kato attended a press conference.
At that time, an incident occurred in which the video was illegally altered to look like a smiling interviewer and was spread on Twitter. In the actual
video, there was no image of the chief cabinet secretary responding with a smile, but because the tweet was published only 30 minutes after the press conference, few people suspected it, and it spread all at once.
As a result, a large number of critical comments such as “unscrupulous” and “unforgivable” were gathered, and it became a big problem. It was an incident that suggested that the evolution of deepfake technology is not only capable of creating sophisticated images and videos, but also that it is a big problem that it can be published easily and quickly.
1.3 Papers on breaking eKYC
A paper published by a Hitachi research group in June 2021 attracted a great deal of attention among those involved.
The paper technically proved that the eKYC system used for personal authentication online could be breached by deepfake technology, and sounded an alarm bell to security officials.
Originally, at counter services such as banks, personal authentication was performed by a method called KYC (Know Your Customer), which compares the face of the person with the ID card. eKYC (electronic Know Your Customer), which is completed online, was legally permitted as a formal identity verification procedure by the revision of the Enforcement Regulations in November 2018.
After that, under the COVID-19 situation, the need for non-face-to-face counter services increased and spread rapidly, so this paper had a great impact on the people involved.
1.4 Fake video detection technology
As deepfake technology becomes more sophisticated year by year, it is becoming more difficult for humans to judge whether a video or image is genuine or fake. On the other hand, technology to detect content created by deepfakes has also been developed to counter deepfake technology. Here, we introduce an overview of fake video detection technology and some advanced efforts.
1.4.1 Overview of detection technology
Fake videos created by deepfake technology often contain unnatural parts, joints in synthesized parts, and other unnatural points. Such unnatural points are called “artifacts” and are used as clues for determining whether a video is fake or not.
Methods for detecting fakes using artifacts can be roughly divided into 1) methods of manually creating logic for automatic detection using artifacts that humans can see, and 2) deep learning technology. There are two methods of automatically detecting artifacts using , etc., and using them to detect fakes.
The following artifacts are known to appear in fake videos.
- Focusing on discrepancies (contradiction) between lip movements and speech[5]
- Loss of eye color or light reflection, partial loss of eyes or teeth [6]
- A method focusing on facial expressions and head movements [7]
- Head movement: Comparison of 3D movement calculated using facial features only and 3D movement calculated using full face features[8]
- Facial expressions: Cheek lift, nose wrinkles, mouth stretch [9]
- Blink pattern, frequency [10]
In addition to the above method of focusing on artifacts, a method of focusing on head movement and comparing 3D motion calculated using only the features of the face and 3D motion calculated using the features of the entire face [49] ], facial expressions, and methods focusing on head movements [48] are known to be effective in detecting fakes.
Next, I will introduce two examples of methods for extracting features that automatically become artifacts using deep learning technology. The first is based on the assumption that the models used to transform faces are often limited in resolution and can only be output at a specific resolution (eg 256 x 256).
In reality, this assumption (restriction) is correct in many cases, and when faces are replaced, traces of the replacement inevitably remain due to post-processing. In order to learn such traces to a deep learning model (ResNet), etc., we sample data about the face area and its outer area, and discover artifacts that are effective for detecting fake videos. This method also performs well.
In addition, methods using invisible feature amounts are also being researched. The technology was originally created to prevent crimes by analyzing data that terrorists hide in images and sounds, but it is also used as a deepfake detection technology.
1.4.2 Microsoft Video Authenticator
Microsoft Video Authenticator is software developed by Microsoft in 2020 for fake video detection. Analyzes images and videos to detect fading and grayscales that are difficult for the human eye to detect due to deepfake technology, and calculates a confidence score to determine whether deepfake technology has been edited. do. Based on this score, it is possible to judge whether it is a fake video [11].
1.4.3 Deep Fake Detection Challenge
In September 2019, a project called DeepFakeDetection Challenge (DFDC) was launched with the cooperation of major IT companies such as AWS, Facebook, and Microsoft, as well as academic experts [12].
The aim of the project is to create technology that will help engineers and researchers around the world detect manipulated media such as deepfake technology. It was held as a competition in a service called “Kaggle” where engineers and researchers from all over the world gather to compete in machine learning and data science technology[13].
The competition kicked off in December, eventually attracting over 2,000 participants to train and validate their models using a unique new dataset created for the challenge. Participants designed a model that could detect fake videos created by AI, and competed on the accuracy of the model.
1.4.4 Pindrop – Fraud Detection for Voice Calls
Pindrop is a security company specializing in voice analytics that analyzes phone voice data to prevent businesses and individuals from being scammed.
Pindrop provides a solution for personal identification and fraud detection that responds to voice communication scams that are becoming more sophisticated year by year[14]. Using deep learning technology, we have established technology to detect voice deepfake technology, and have achieved results[15].
2 Social implementation of generative deep learning
As we have repeatedly explained in this article, deepfake technology and generative deep learning technology, which has greatly contributed to its evolution, are technologies that can be abused depending on how they are used.
But also. It is expected that many more products and services will be created in this technical area in the future. This section introduces the products and services that have already been introduced to the market, and the technologies that are scheduled to be introduced in the near future.
2.1 NVIDIA – Maxine
In October 2020, NVIDIA announced Maxine , a video conferencing platform that utilizes AI technology . According to NVIDIA’s announcement, Maxine internally uses GAN to restore compressed data[17], reducing the bandwidth (communication data volume) required for video conferencing software to about 1/10. can do Instead of shooting and sending video as input, Maxine extracts a set of keypoints extracted from the source video.
Since this data can be expressed as much smaller data than normal video, it is possible to greatly reduce the amount of communication and transmit more frames. On the receiving side, GANs are used to reconstruct the original face image from a set of keypoints.
Technologies like Maxine have the potential to solve the bandwidth-hungry problem caused by the proliferation of online meetings by enabling high-quality video communication even in environments with limited network bandwidth. ing.
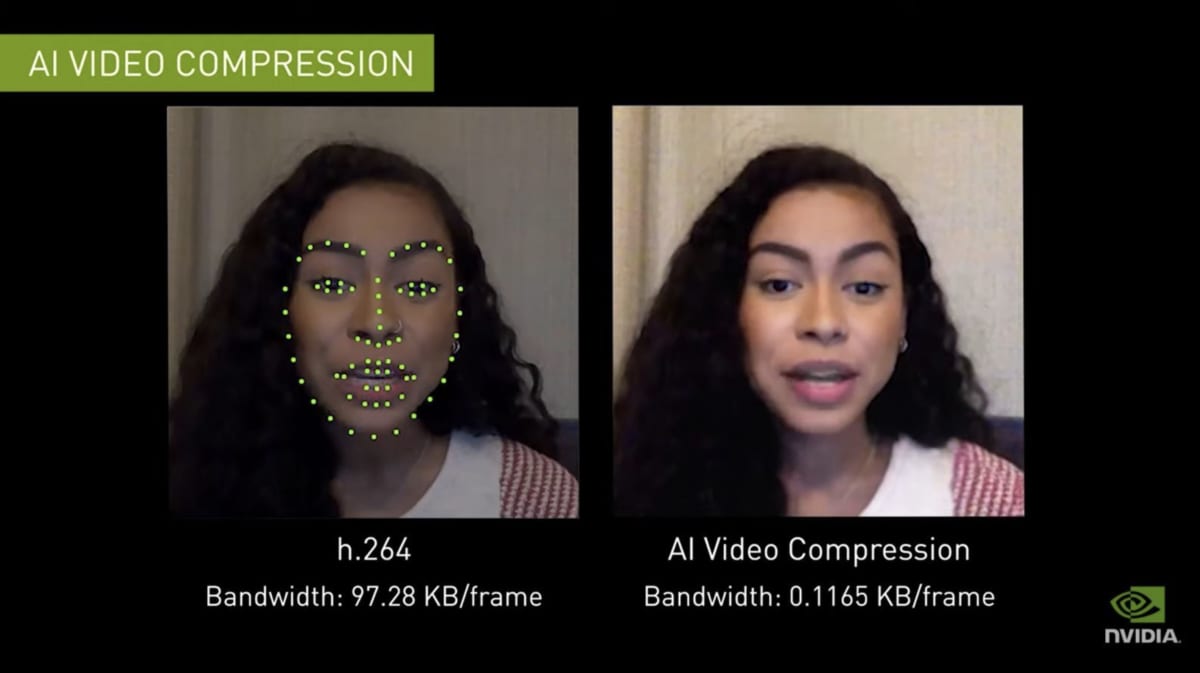
2.2 Descript – Overdub
Descript publishes and provides ” Overdub “, a voice cloning technology that allows a person to speak any content with a one-minute sample voice [18]. It is provided as part of a tool for podcasts called Descript, and is expected to be used mainly for editing part of the audio recorded for distribution and adding audio.
The technology was originally developed by a Montreal-based venture company called Lyrebird, but has now been acquired by Descript and continues to develop the technology as an AI research division.
2.3 Generated Media – Generated Photos
“Generated Photos” is a service provided by Generated Media that allows users to download over 100,000 images of fictional characters generated by GAN. By checking the photograph, it can be seen that the quality of the face image is so high that it is very difficult for a human to recognize that the image has been generated.
A technology for generating a face image of a fictitious person has a high degree of freedom in terms of portrait rights, and thus is expected to have various industrial applications such as generation of avatar profiles and game characters. Internally, StyleGAN is used as a face generation (Entire Face Synthesis) technology.
2.4 Artificial tooth design by GAN
The University of California, Berkeley and Glidewell Dental Lab jointly developed a GAN to design artificial teeth [20]. When creating a new artificial tooth, it is necessary to satisfy many requirements at the same time, such as that the finished artificial tooth should fit the patient’s dentition, have a good bite, and be beautiful.
This makes it a highly specialized and difficult task that would normally be handled by a technician with years of training. Although the introduction of design support tools has reduced the burden of designing artificial teeth, it is still necessary for dentists to evaluate and adjust the design.
With the new GAN-based method, it is possible to automatically design not only from the knowledge obtained by humans, but also considering the space with the opposite tooth.
Designing for the space between the missing tooth and the opposing tooth is a difficult task for dentists, but it is an important factor in achieving a good bite and the final prosthesis. significant impact on tooth quality.
According to published research results, artificial teeth made with GAN technology fit the patient’s mouth better and have a better bite (the red part in the figure is the part that collides with the opposite tooth). ).
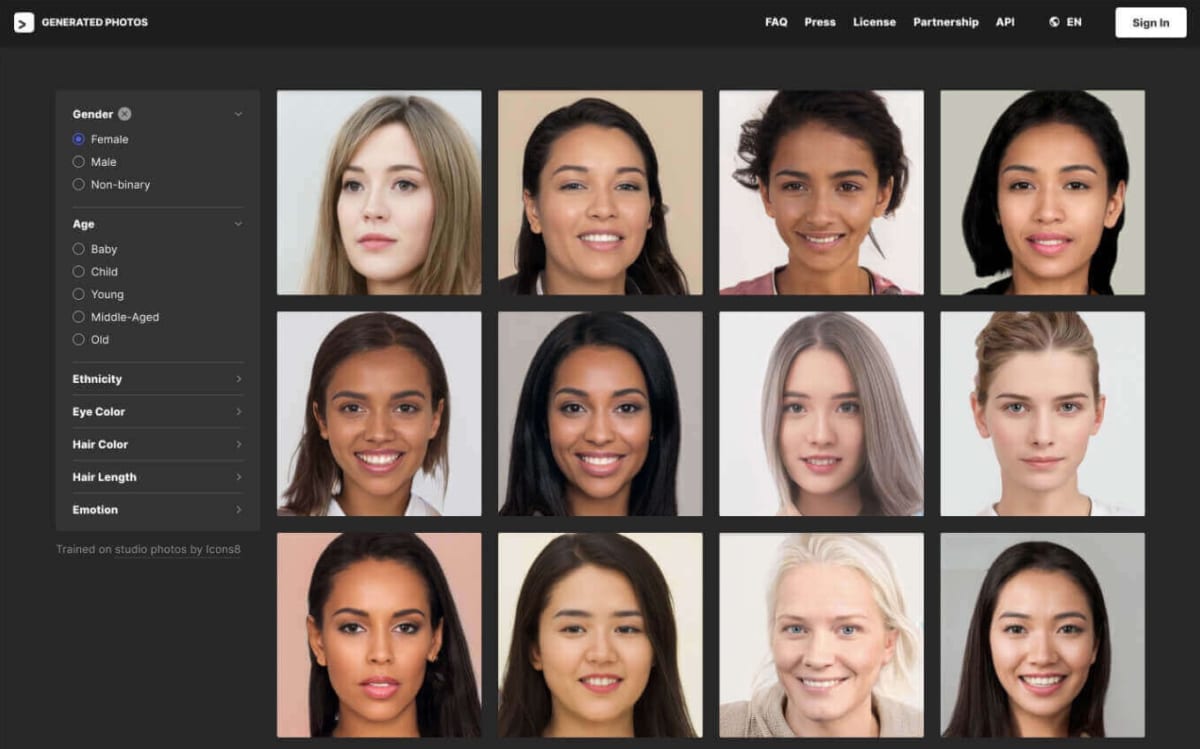
2.5 Application to design and art
Generative deep learning technology is said to have the potential to greatly affect the design and art production process, and we are already seeing more and more works using such technology. In this section, we will introduce some examples of technologies related to design and art that are already familiar to us.
The first example is “PaintsChainer”[21] developed by Preferred Networks. PaintsChainer is a technology that automatically colors monochrome line drawings, and internally consists of a neural network with a structure similar to Pix2Pix.
When considering the problem of generating a color image from an input line drawing, the technical difficulty lies in the fact that the correct answer cannot be uniquely determined. There are a myriad of patterns for coloring, and in many cases it is not a mistake to color a part that is already colored blue into red.
When the correct answer is not uniquely determined in this way, there was a problem that it was difficult to create a model that can obtain a good output with the conventional method. ” and build a model that trains adversarially so that the generator produces more natural colored images.
This technology is used in a service that automatically converts monochrome manga into color and distributes it (PaintsChainer was transferred to pixiv in November 2019 and is now operated as Petalica Paint).
The second example is “GauGAN” [23]. GauGAN is an application developed by NVIDIA that can generate photographic-like landscape images simply by inputting a rough layout. It is possible to control what kind of scenery image is output, and it is possible to generate images while changing the style, such as sunset or beach, from one layout.
NVIDIA reported that 500,000 images were created in one month when it was released to the public in 2019. Internally, a GAN model called SPADE [24] is used. It is expected that it will be possible to create a production process that allows the user to concentrate on creating the foreground part while automatically creating the scenery part of various artworks.
The images that can be created are limited, and there are issues with practicality, but in the future, as this technology becomes more sophisticated, a wider range of production and various video production processes will become more efficient. etc. is expected.
2.6 Application to industrial product inspection
Most generative deep learning technologies aim to create data that closely resembles the distribution of real data (data close to the real thing), but the internal processing includes logic to determine whether it is close to the real data.
It is included. By making good use of this, it is possible to quantify and judge whether or not the data is close to the real data. The technology for discovering data with characteristics that are different from the characteristics of data that has been observed so far is called ” anomaly detection ” and can be used in the inspection process of industrial products.
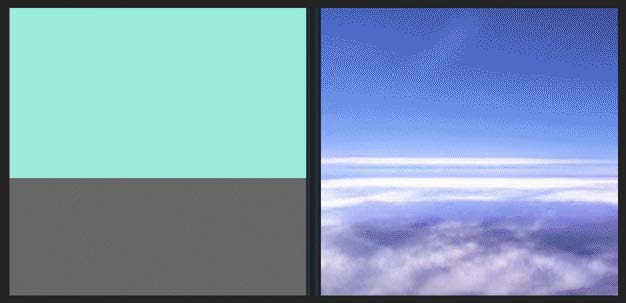
AnoGAN is a pioneering technology among methods that apply GAN technology to anomaly detection[25]. In the manufacturing process of products, there are many cases in which the number of products with abnormal characteristics (such as scratches and defects) is extremely small compared to the number of normal products, and in many cases it is difficult to create data on abnormal products.
In this way, data that contains a very small percentage of the data you want to detect is called “imbalanced data”, and it is not possible to find data with abnormal features from such data with ordinary machine learning methods. is a difficult problem.
Therefore, there is a need for a technology that can determine whether a part is normal or abnormal based only on the data of normal parts that can be easily obtained in large quantities. can.
In AnoGAN, we first train a GAN model using a large amount of data only for normal products, and by grasping the patterns and characteristics of normal products, we create a model that can generate data that is close to the real thing (normal product). to build. Next, the data (image) to be inspected is input, and the image is reconstructed through the previously trained GAN model.
At this time, data containing patterns that have never been seen before, such as scratches and defects, cannot be generated well, so by taking the difference between the original image and the generated image, it is possible to determine whether the product is abnormal.
It is possible to detect areas that are highly likely to contain abnormalities, and to calculate numerical values such as the degree of abnormality.
Anomaly detection technology using generative deep learning is a highly versatile technology, and is expected to be applied in various fields other than inspection work of industrial products. For example, it is expected to be applied to the discovery of diseases in medical images and the detection of malware in cyber security.
Summary
This whitepaper describes deepfakes, which have become one of the most serious threats in AI crime in recent years, and the underlying technology. Deepfake technology is becoming more sophisticated year by year, and it is becoming possible to generate high-quality images that are difficult for the human eye to distinguish.
In fact, there have already been a number of malicious incidents around the world, and social concerns continue to grow. In addition to images and videos, technology for cloning audio is also evolving.
However, at the same time as the development of technology to detect fakes, such as Microsoft Video Authenticator, is also progressing, services and products are being developed to prevent damage caused by fake images and sounds.
It is expected that the development of deepfake technology will continue to evolve in the future, but along with detection technology, it is thought that it will be important to create a social foundation and a mechanism for responding, such as legal development and moral education.
On the other hand, generative deep learning technology, which has been related to the evolution of deepfakes, is a technology with great potential depending on how it is used, and has the potential to bring about major changes in various industries.
In fact, various basic technologies and products have already been developed, such as data compression/decompression, design/art applications, industrial applications, and anomaly detection applications.
In the future, AI technology is expected to change the structure of industry in various fields and bring about changes in society. At that time, it is important to understand what you can do and what you can do, rather than having vague fears and expectations based on a superficial understanding.
Isn’t it an attitude to take advantage of it? We hope that this white paper will serve as an opportunity to learn about such technical limits and possibilities.