What is multi-omics analysis? Introducing reasons for attention and examples
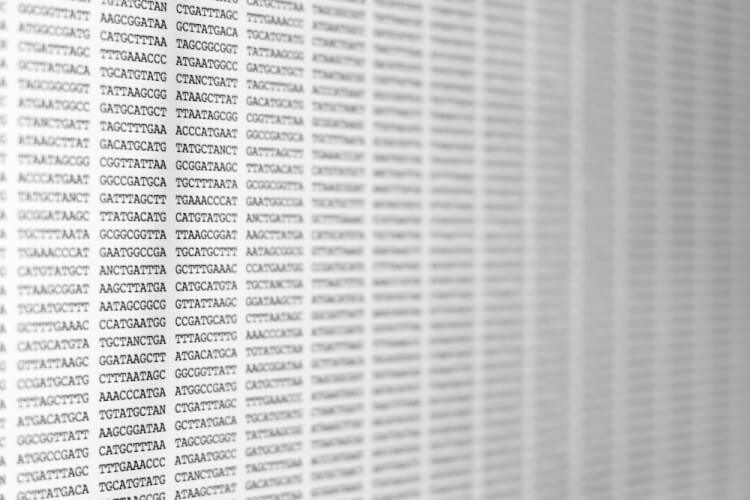
Table of contents
- What is multi-omics analysis?
- Understand the causes of diseases at the genetic level
- Background to the focus on multi-omics analysis
- It has become possible to accumulate large amounts of biodata such as genes.
- Advances in AI technology
- Examples of multi-omics analysis
- Research on biomarker molecules related to aging and diseases
- summary
What is multi-omics analysis?
Information that comprehensively summarizes molecules existing in the body is called “omics,” and analysis using this information is called omics analysis (or omics analysis).
Furthermore, analysis that uses various omics information and spans multiple omics is called “multi-omics analysis.” In recent years, omics information has been attracting attention as it can provide new medical and biological knowledge that could not be obtained from just one type of omics information.
For example, investigating “disease risk/genetic diseases” and “cancer-specific gene mutations” by DNA analysis, investigating “cancer-specific gene expression abnormalities” by RNA analysis, and investigating “cancer-specific proteins” by protein/peptide analysis. “abnormalities” and “cancer-specific metabolite abnormalities” through metabolite analysis.
Although these analyzes are useful for investigating specific information, it is important to analyze them in an integrated manner in order to make use of more personalized medicine and elucidate the characteristics of cancer.
Therefore, by performing multi-omics analysis, it will be possible to elucidate the characteristics of cancer and implement personalized medicine, which will be useful for disease prediction and treatment. This analysis is also useful for pre-symptomatic medicine, and is said to be useful for pre-symptomatic diagnosis and preventive treatment.
Although multi-omics was originally expected to be effective in theory, it has not been realized easily due to technical constraints. The data was uneven, too complex, and difficult to analyze using human knowledge alone.
As will be explained later, the development of IT technology and the accumulation of large amounts of information have led to the practice of multi-omics analysis in recent years.
Understand the causes of diseases at the genetic level
As mentioned above, omics analysis refers to the act of comprehensively analyzing biomolecular information (genome, etc.).
A genome is a block of DNA that contains genes that function like a blueprint for a cell. In other words, by elucidating the structure of genes, we can learn why visible biological characteristics such as hair color and height are expressed and how those characteristics appeared. is.
This is not limited to just looking at external characteristics, but also leads to finding the causes of symptoms of diseases such as cancer. If we can understand the fundamentals of disease manifestation, we will be able to provide more precise treatment and prevention.
Background to the focus on multi-omics analysis
Previously, there were technical problems with algorithms and data processing, which prevented analysis from proceeding even if medical and biological data were registered in public databases. In other words, the data was not being fully utilized.
However, in recent years, as explained below, it has become possible to accumulate large amounts of biodata and advances in AI technology such as deep learning have led to multi-omics analysis attracting more attention. I did.
It has become possible to accumulate large amounts of biodata such as genes.
Before reaching the current level of technology, decoding the human genome required a huge amount of money, exceeding several hundred billion yen. However, it is now said that the cost of decoding the entire human genome sequence for one person has decreased significantly, and the data generation speed has decreased to about one-thousandth of a factor.
Additionally, biobanks, research projects that track the onset and course of diseases over the long term, are progressing rapidly, and a large amount of genomic information is being accumulated. This has facilitated the elucidation of the genetic factors and molecular mechanisms of diseases, and has improved the level of individualized preventive medicine.
Furthermore, the advent of mobile health is also contributing to the accumulation of large amounts of biodata. With the rapid development of smartphones, the Internet, etc., mobile health, such as wearable biosensors, is becoming more popular. By measuring individual data continuously for 24 hours, it is possible to generate time-series data, and by comparing it with other personal data, it is possible to explore the characteristics of each individual and the possibility of disease onset.
Additionally, as big data has become easier to process, applications that can improve business efficiency are increasingly being developed in all industries.
Advances in AI technology
In the previous section, we mentioned that the technical development of big data is progressing and the accumulation of data is progressing, but the huge amount of data collected is meaningless unless it can be processed appropriately and efficiently. It is important to conduct analyzes that yield useful information.
Another factor that can be said to be related to the increased attention to multi-omics analysis is the evolution of AI algorithms. In particular, deep learning is strongly related to improving the efficiency of genome analysis. It is no exaggeration to say that the development of deep learning is at the core of the development of AI technology.
Deep learning further develops machine learning and allows machines to perform autonomous learning, discovering certain characteristics from big data, and creating rules for themselves.
In recent years, the number of situations where AI is used has increased. For example, through joint research with the Institute of Medical Science, the University of Tokyo, Fujitsu Laboratories has developed AI technology to make cancer genome medicine more efficient, and is actively working on various cancer genome medicines that utilize AI. It is working. We can expect that AI technology will continue to develop and be used in an increasing number of situations.
Examples of multi-omics analysis
Multi-omics analysis is attracting attention due to the accumulation of big data and advances in AI technology, and we will introduce examples of this analysis being used in practice.
Research on biomarker molecules related to aging and diseases
This research was conducted by the National Center for Geriatrics and Gerontology Research Institute, and used blood omics information to search for biomarker molecules related to aging and diseases.
We conducted multiple analyzes using blood from patients and healthy individuals, and conducted molecular searches for Alzheimer’s disease and analysis of blood molecules associated with aging. As a result, microRNAs whose expression changes in disease groups were detected, and changes in metabolites were confirmed. Additionally, omics analysis of the Alzheimer’s disease model mouse brain revealed that changes in 17 types of microRNAs were detected.
summary
Advances in IT technology will continue to enable things that were previously impossible to achieve, such as multi-omics analysis, and furthermore, it will be used in business applications. One of these, Microsoft Dynami CS 365, will enable integrated management of customer management and applications, and is expected to further optimize business operations.